A post about AI after taking an MIT course and a bit of my views on AI & AECO
- Iria Carreira
- Jul 17, 2024
- 2 min read
During April and May, I enrolled in an MIT course focusing on AI and ML for product design and development. My familiarity with machine learning (ML) came from my time at CASA, urban analytics, and various projects I have worked on. However, the intricacies and details of AI models remained very confusing for me, and I wanted to get a deeper understanding into these unknown aspects, a bit of "I wanted to know how much I didn't know".
In the context of AI and the Architecture, Engineering, Construction, and Operations (AECO) industry, I believe the key lies in PDF data rather than Building Information Modeling (BIM) data. This perspective might seem unconventional, but most historic and current AECO information is stored in PDFs, whether smart or otherwise. AECO is an industry where precision is fundamental, and detailed drawings (e.g., 1:5 scale) are crucial. In my humble opinion, the future leader in AI AECO will be the company that can extract intelligence from these detailed documents. Even cooler, companies that enable model creation from existing data will drive the implementation of AI in AECO. My goal with the course was also to understand what type of technology models could accomplish this.

Whenever I encounter AI-related posts on LinkedIn regarding AECO, they often list ten AI tools in a very general manner without explaining how specific AI models are beneficial for particular applications in our industry. As someone who is curious about innovations and future trends, I have learned that the first step in adopting any new trend is to comprehend the unknown. This reminds me again of the Socrates quote: "I only know that I know nothing."
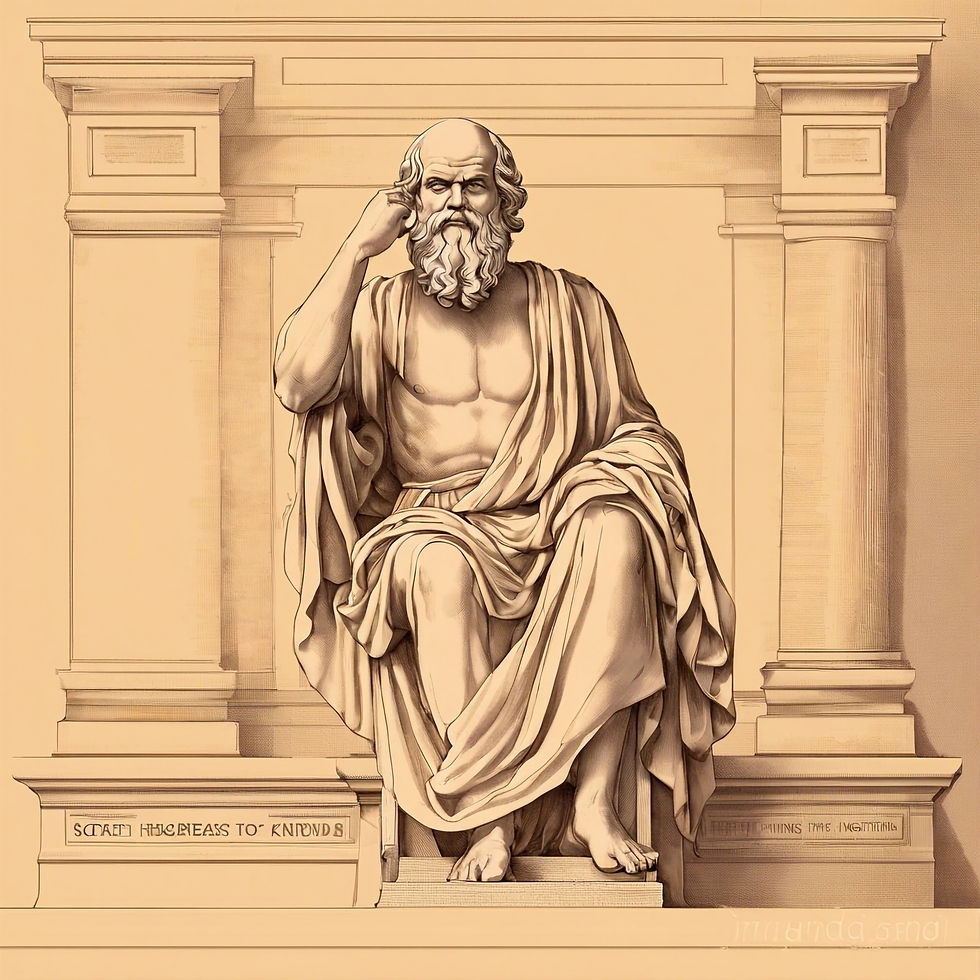
So to share a bit from my MiT course, and rather than perpetuating the unhelpful trend of listing "100 products that you can use and they do xyz," I have decided to take a different approach. Here are the themes I aim to learn more about in the upcoming year after completing my course:
Classifiers in Machine Learning: Understanding how classifiers work and their applications in various scenarios.
Supervised, Unsupervised, and Reinforcement Learning Methods: Exploring different machine learning methods and their use cases.
Neural Networks: Delving into convolutional neural networks (CNNs) and deep neural networks (DNNs) to understand their architectures and applications.
Combining AI and Human Intelligence: Investigating how AI and human intelligence can be integrated to create super minds.
Human-Computer Interaction (HCI): Studying the interaction between humans and computers to design more intuitive AI systems.
Refreshing my maths and expanding my math knowledge: this sounds basic but most ML and foundational pieces of AI required to learn maths. There is no escape.
If you asked me where to find more information about these subjects, I still have access to some documentation from the course, but mainly I have been using resources from IBM videos. Then two recommendations from some folks in my engineering team the coding train Youtube Channel, and keep reading The Little Book of Deep Learning until I actually get it. If you have any good resource for learning more complex maths as an adult, drop it in the comments.
Yorumlar